We started ControlTheory because we were frustrated and disappointed with the current state of observability and how it has evolved over the last 10 years. As co-founders and engineers, we’ve worked in this space for most of our careers, and in talking to hundreds of organizations, we were left with a fundamental observation:
Observability is broken and needs to be fixed. Our honest assessment is that observability has devolved into dumb pipes sending massive telemetry streams into bloated datastores.
The end results are well-documented and discussed: escalating costs, proprietary closed platforms, vendor lock-in, data silos, diminishing ROI, as well as rising MTTR and desultory root cause analysis. Not surprisingly, it turned out that we were not the only ones frustrated and disappointed. If observability was going to rise to meet the challenges of the next decade, something needed to change.
It Started With a Feedback Loop
ControlTheory began with a simple thought: what if we added feedback loops across the observability supply chain? From that basic idea, the impossible becomes possible:
- Collection gets smarter and new analytics thrive
- SREs, platform teams and developers have more control and insight
- The equation flips from “all your data” to “just the data you need, when you need it, and for whom”
- Observability intelligence becomes less centralized and redistributed across the observability supply chain, from code to collection to cloud
- New KPIs become more affordable and accessible
- A new generation of incident management and root cause analysis tools emerge driven off a more intelligent and adaptive data platform
- The whole process becomes more intelligent and more modern
In a nutshell, observability elevates and evolves.
Feedback loops brought back memories of the basics of Control Theory. We’re all engineers – I was a Mathematical Science major with a Masters in Electrical Engineering. We are well-trained in the theory of control systems and feedback loops. Controllability is a peer to observability, and feedback loops are the foundation to achieving stability in unstable systems. Thus, a new startup ControlTheory was formed.
Observability and Controllability
Over the last decade, observability innovation has brought together a unified best practice to manage logs, metrics, and traces across the development and operational lifecycles. But observability in its current form has stagnated. Consolidation has replaced innovation. Organizations feel frustration. Something is missing to balance out and elevate observability to meet the challenges of the next decade. Controllability is that logical missing piece.
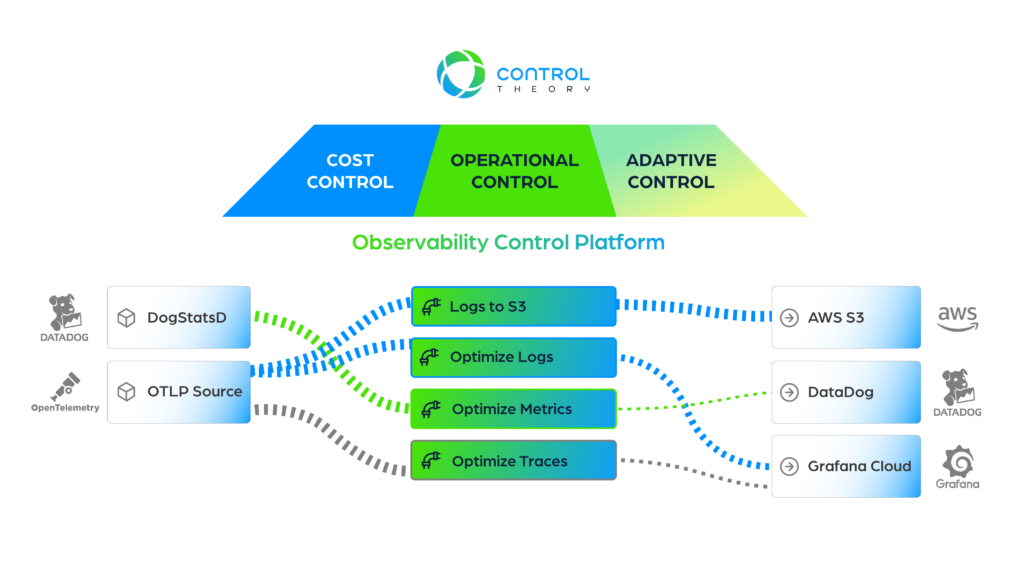
Controllability is the ability to stabilize and control unstable systems through feedback loops and input signals for continuous improvement. A feedback loop uses output as input to create a continuous cycle of improvement to self-stabilize and self-regulate. While Controllability is the ability to control the state of the system by applying specific input signals, observability is a measure of how well internal states of a system can be inferred from knowledge of its external outputs. In control theory, the observability and controllability of a linear system are mathematical duals, based on the amazing work from Rudolf Kalman the father of modern Control Theory.
ControlTheory: Regain Control of Your Observability
Controllability enables organizations to regain control of their observability through:
- Cost Control: detecting spikes, reducing metric cardinality, intelligently rerouting and filtering logs and traces, avoiding vendor lock-in, using open standards versus closed proprietary tooling
- Operational Control: sharpening root cause and anomaly detection by increasing signal, decreasing noise through intelligent sampling, illuminating current telemetry through observability “Meta-Metrics”
- Adaptive Control: Elastic Telemetry Pipelines use dynamic feedback loops to govern through policy for continuous improvement and adjustment, auto-scaling telemetry up or down for new releases and iterative troubleshooting without having to change your code
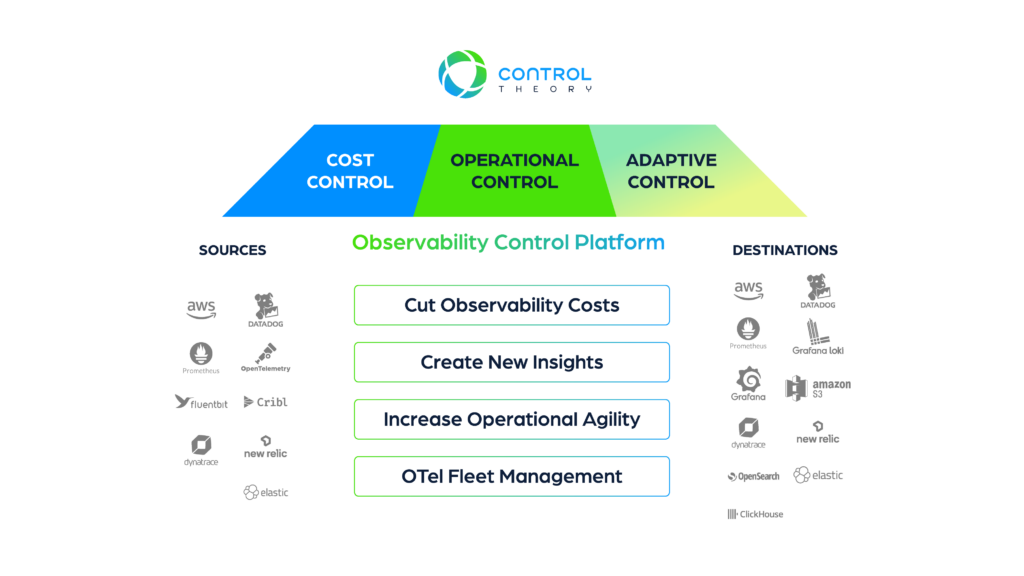
Four Key Controllability Building Blocks
For ControlTheory, a controllability strategy formed around 4 key building blocks: open collection and instrumentation, a control plane pattern, adaptive feedback, and asking “why?” That last tenet – asking why – is critical to raising observability to the next level. Rising observability costs are painful, but they are a symptom of a more foundational issue. Why are we observing our infrastructure and application performance in the first place? The end game of observability is not to observe but to prevent and solve problems, reduce MTTR, accelerate root cause analysis, and unearth critical new business KPIs.
1. OpenTelemetry: the First Observability Disruption Domino
OpenTelemetry is already beginning to disrupt observability as we know it. Change starts from the bottom up: instrumentation and collection. If these are proprietary and fixed to your observability vendor then you are locked in as the observability vendor effectively “owns” the telemetry data that is generated, stored, and any analytics performed on it. This is an all too familiar problem when it comes to (annual) renewal time. The emergence and maturation of OpenTelemetry from the CNCF – enables open, broad, democratized, and programmable collection. It enables any source, any destination, and user choice – so you are the one that now “owns” your telemetry data, not just for the sake of it, but for the additional capabilities and insights that you can unlock from it. Open and vendor-neutral will win over proprietary.
2. An Open Control Plane for Scalable Control
Having architected, and built Oracle Cloud’s Kubernetes service (OKE) and additional cloud native services, we knew that the “data plane – control plane – management plane” pattern was essential for a scalable cloud native service. OTel needed a viable, open control plane to control and manage its open data plane. By focusing on the control plane, organizations can control their own data and maintain agency over their information. Just like a traditional control system (e.g., heating and air conditioning) needs control points, we need to be able to flexibly control our telemetry so that it can evolve from one-way, dumb pipelines to a 2-way system that controls our telemetry to achieve a given desired state.
3. Adaptive Feedback Loops: Elastic Telemetry Pipelines
Feedback loops in all forms – auto-tuning, reinforcement learning, reflection, human-in-the-loop, and iteration – will be essential to elevate the next wave of observability. An elastic telemetry pipeline improves on the current state of static telemetry pipelines. If our infrastructure and applications are elastic, orchestrated, and can auto-scale, why settle for static observability that pumps data out without regard to any built-in intelligence, policy, or control. WIth elastic telemetry pipelines, common patterns of baselining and auto-threshholding that have been table stakes for generations of centralized application performance and infrastructure monitoring tools can move into the control plane. This frees up new analytics based on causality, inference, agentic, and other emerging AI tech to blossom, flourish and thrive.
4. The Why of Observability
Why am I collecting all this data? Why do I need to store all these logs? Why can’t we find the root cause of this issue? Why did it take us three days to track down that problem? If you start with the why’s, observability objectives become clearer. Observability today relies too much on the philosophy “collect and store everything, ask why later.”
ControlTheory Origin Story Summary
Transformation requires agility and adaptability. Observability today is too rigid and brittle for organizations to actively control their destiny to support whatever their business requires: migrations, consolidations, improvements to RCA and MTTR improvements, security and compliance, and the introduction of new AI technologies. ControlTheory brings the power of the feedback loop to observability, driving agility, adaptability, and transformation.
press@controltheory.com