AI SRE Tools Need Better Data
Home / Solutions / Control Your Datadog Bill / Deliver Curated Data to AI SRE Tools
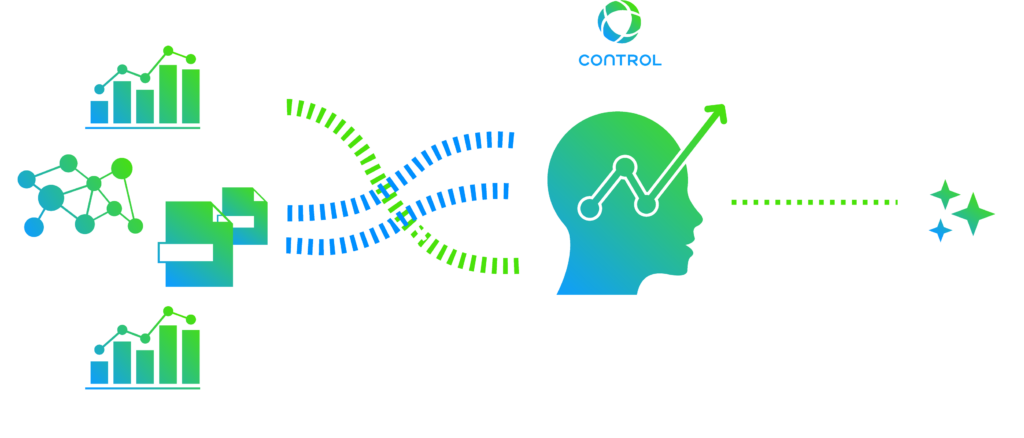
Deliver Curated, Clean Telemetry to AI SRE Tools
Problem – AI Tools Need Clean Telemetry, Garbage In Garbage Out
The field of AI-powered Site Reliability Engineering (AI-SRE) is rapidly evolving, promising intelligent systems capable of detecting, diagnosing, and even remediating issues across complex, distributed environments. From causal graphs to automated root cause analysis (RCA), the vision is undeniably compelling.
However, as Andrew Mallaband highlights in his article “Value-Driven Observability: Aligning Data with Business Impact,” these advanced tools are heavily dependent on high-fidelity telemetry — comprehensive, clean, and context-rich data. Without this foundation, even the most sophisticated AI-SRE solutions struggle to deliver on their promises with access to curated, clean telemetry, especially when powered by incomplete or mismanaged observability data from platforms like Datadog or OpenTelemetry.
The AI-SRE Promise vs. Reality
AI-SRE tools aim to transform how we manage complex systems by leveraging artificial intelligence to enhance reliability and efficiency. But there’s a fundamental issue: data quality. As Mallaband emphasizes, the effectiveness of AI-SRE is directly tied to the quality of the telemetry it processes — including logs, traces, and metrics sourced from systems like Datadog and enriched through OpenTelemetry.
When telemetry data is noisy, incomplete, or inconsistent, several critical problems emerge:
- Inaccurate Root Cause Identification: AI models struggle to pinpoint true root causes amid low-quality data.
- Erosion of Trust: False positives and missed detections undermine confidence in AI recommendations.
- Wasted Engineering Time: Teams waste hours filtering noisy telemetry, negating efficiency gains promised by AI-SRE.
This ‘garbage in, garbage out’ scenario prevents AI-SRE tools from reaching their full potential and can lead to increased Datadog billing for data that offers limited actionable value.
Solution – ControlTheory Elevates Data Quality for AI-SRE
ControlTheory addresses this foundational challenge by improving telemetry quality before it reaches AI-SRE tools. This includes logs, traces, and Datadog metrics — managed through smart observability pipelines.
The solution operates on three key principles:
- Unified Telemetry Pipelines: Consolidate data into a unified observability pipeline compatible with OpenTelemetry and Datadog agents to eliminate silos and ensure consistency.
- Intelligent Data Processing: Filter out high-cardinality noise and enrich key signals before the data reaches your AIOps platform, preventing expensive over-ingestion in tools like Datadog.
- Granular Visibility and Control: Teams gain observability into telemetry flows, enabling fine-tuned decisions that balance cost, performance, and reliability.
The Outcome: Transitioning from Reactive to Proactive Reliability
Integrating ControlTheory into your AI-SRE and observability strategy enables:
- Reduced MTTR: With better root cause precision, incidents resolve faster.
- Proactive Issue Resolution: Detect issues before they reach customers.
- Strategic Resource Allocation: Engineers focus on what matters, guided by high-quality insights.
This reduces Datadog billing waste while improving how data flows into AI-driven diagnostics — resulting in smarter, leaner reliability operations.
Ready to Transform Your AI-SRE Strategy?
‘t miss out on the opportunity to unlock the full potential of AI-SRE for your organization. Schedule a consultation with ControlTheory today to:
- Audit your existing telemetry pipelines and Datadog logging setup
- Implement OpenTelemetry-based data processing and enrichment
- Create a roadmap for scalable, cost-efficient AI-SRE adoption
Take the first step toward observability that fuels intelligent, trustworthy automation.